Embracing AI for Competitive Advantage in the Financial Industry
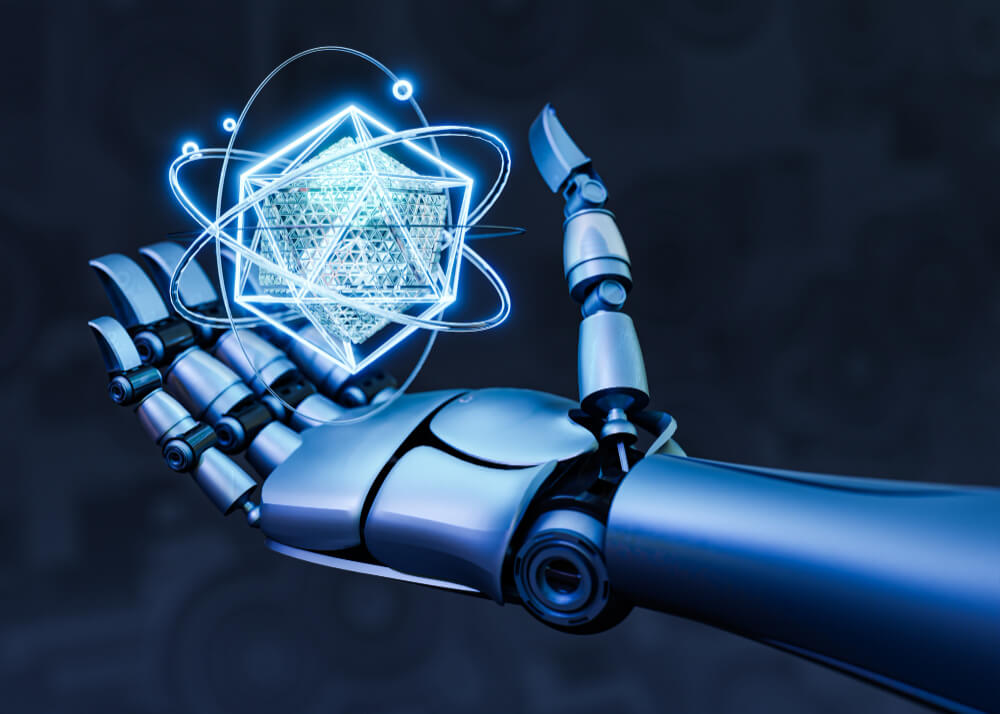
Amid escalating competition from industry players, financial institutions and banks have long been attempting to leverage Artificial Intelligence (AI) to augment client experiences and sustain their market position. Despite these efforts, results have been marginal, primarily due to the limited enterprise-wide implementation. There is untapped potential for AI in banking, predominantly in revenue optimization, yet it has not been fully exploited by most firms.
Considering the rapid advancements in AI and GPT technologies and the extensive capabilities these tools offer, financial institutions possess an invaluable opportunity to unlock AI’s full potential strategically and practically.
By committing to a set of key principles, industry leaders and executives can engineer operational advancements, converting incremental gains into substantial enterprise-wide improvements:
The first and most important step is to recognize the value that AI adds to the process of operational improvement. Financial institutions must have a thorough knowledge of both the advantages and disadvantages of this disruptive technology.
Organizations may create attainable objectives and foresee a future of digital transformation and increased competitiveness through AI solutions specific to the banking sector by encouraging cooperation between business and technical teams.
Financial firms may use the 10-20-70 model to properly examine the benefits of AI. This methodology entails devoting resources to three essential areas: machine learning model development, data and technology integration, and business process reform overhaul.
The cautious selection of AI applications is a critical element in this approach. Even within a single category, raw data quality has a considerable influence on the performance of machine learning algorithms. Collaboration with business solution professionals enables financial institutions to precisely adapt data inputs, resulting in more precise and useful insights.
Financial organizations must combine external data sources alongside their internal data to preserve flexibility in an ever-changing environment. Using just internal data may restrict an organization’s agility. Financial institutions may better analyze changes in the operational environment by integrating extensive, accurate, and relevant external data.
Another essential necessity is to simplify complicated AI models into manageable submodels that correspond with business logic and restrictions. Financial organizations boost their chances of successful AI adoption by addressing particular problems with reduced AI models.
It is critical to emphasize machine learning’s capacity to help targeted business decision-making and bring value beyond prediction accuracy when evaluating it. Optimization algorithms are critical in offering decision-making tools that are linked with corporate objectives such as profit maximization, cost minimization, and lead time optimization.
An AI system’s accuracy, dependability, and practicality must all be considered while evaluating it. Conducting sensitivity testing on a wide variety of variables aids in determining the system’s adaptability to future changes.
Collaboration between data scientists and business domain specialists is required for successful achievement of these goals. By forming multidisciplinary teams that combine technological skills with operational insights, financial institutions may fully realize the potential of machine learning.
Financial institutions and banks may place themselves at the vanguard of AI integration by embracing these specific principles, generating operational efficiencies, and enjoying the full advantages of this disruptive technology.